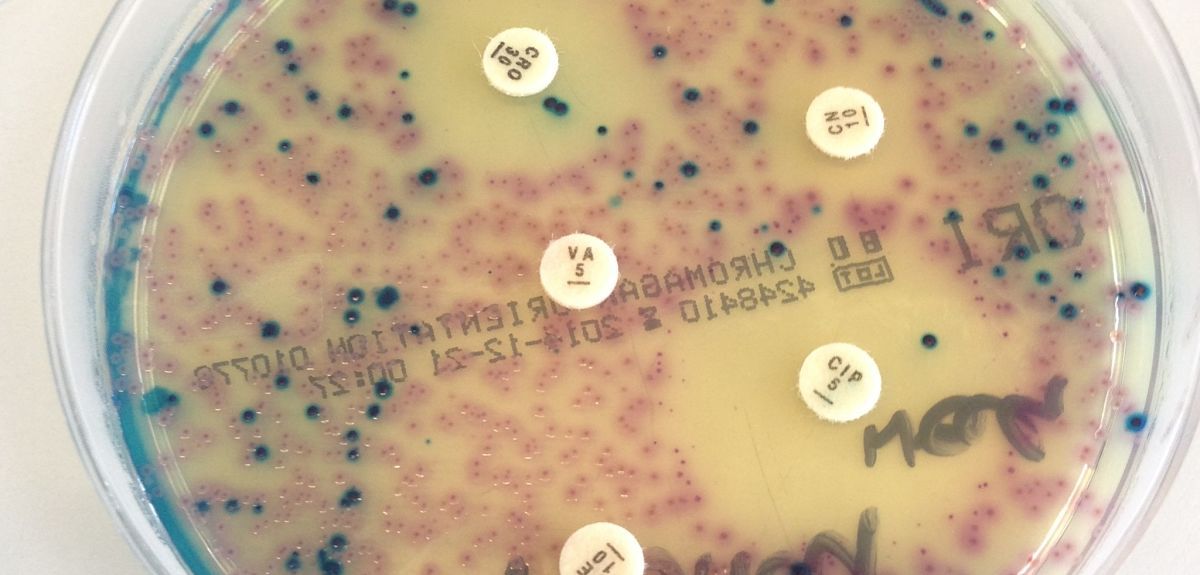
Image Credit: Dr Nicola Fawcett, Nuffield Department of Medicine
Resistance is futile
A new computational model helps to find innovative ways to tackle the dangerous problem of antibiotic resistance in bacteria. Doctoral student Dan Nichol explains his research.
Antibiotic-resistant strains of bacteria are currently causing an annual worldwide death toll totalling hundreds of thousands of lives. A recent report from the Review of Antimicrobial Resistance, commissioned by the government, suggests that this number could reach 10 million by the year 2050. This rapid increase in the rate of antibiotic resistance has been coupled with a drastic slowdown in the discovery of novel antibiotic compounds. It is becoming ever clearer that this crisis cannot be solved simply by discovering novel antibiotics; instead we must find new uses for existing drugs in treating highly-resistant disease.
This crisis cannot be solved simply by discovering novel antibiotics; instead we must find new uses for existing drugs in treating highly-resistant disease.
Traditional medical wisdom is that to effectively treat infections we should prescribe the most effective drugs, at the highest tolerable dose, for as long as is needed to clear the infection. However a new paradigm, known as adaptive therapy, which has emerged from mathematical modelling of the treatment of bacterial infections, viruses and cancer suggests that this strategy may be driving resistance.
By considering disease from the Darwinian perspective, where treatment imposes selective pressure and drives the evolution of resistance, mathematical modelling (coupled with biological experiments and clinical observation) suggests that the optimal treatment strategy may be to prescribe multiple drugs, in sequence or in combination, to pre-empt or exploit evolution.
Using a simple computational model which encodes the population dynamics of an evolving bacterial population as a Markov chain, Daniel Nichol and Jacob Scott have built a tool capable of predicting sequential adaptive therapies for E. coli which reduce, and in many cases entirely prevent, the risk of highly resistant disease emerging.
To design these adaptive therapies it is first necessary to predict how a population of bacteria will evolve. Recently published empirical measures of the fitness landscapes of E. coli under fifteen different antibiotics have made these predictions possible. A fitness landscape is a mapping which assigns to each possible bacterial genotype an associated level of resistance, or fitness, to a given drug. The landscape metaphor, first introduced by Sewell Wright in the 1930s, is a visualisation of this mapping as a surface on which an evolving population ‘climbs’ uphill.
The order in which drugs are given will have a significant impact on the final state after evolution.
By considering the relevant bacterial genotypes as the vertices of a weighted directed graph, where the edge weights encode the probability of a change in the population genotype, this ‘uphill climb’ in the fitness landscape can be reduced to a biased random walk on a graph. By encoding this random walk formally as a Markov chain, the complex process of evolution within a population of bacteria exposed to an antibiotic is reduced to a single matrix multiplication. The immediate consequence of this model is that, as matrix multiplication is non-commutative, the order in which drugs are given will have a significant impact on the final state after evolution. This observation leads to a natural question: are some orderings better than others?
In their work, published in PLOS Computational Biology, Daniel and Jacob show that the answer is ‘yes’. Using the recently published landscapes for E. coli, the research demonstrates that it is possible to prime the disease population using sequences of one to three antibiotics such that resistance to a final antibiotic cannot emerge. This finding suggests a new treatment strategy in fighting antibiotic disease: adaptive therapies which use sequences of drugs to steer, in an evolutionary sense, a disease population to a configuration from which it is both readily treatable but also from which resistance cannot emerge.
As well as demonstrating the possibility of adaptive, sequential therapy for treating highly-resistant bacterial infections, Daniel and Jacob also provide a cautionary warning regarding current clinical practice. When antibiotics are prescribed in sequence, as is often the case in treatment of H pylori, Hepatitis B or the transition from broad to narrow spectrum antibiotics, no guidelines presently exist to specify the order in which drugs should be given. Instead this decision is left to the clinician’s personal preference
Over 70% of drug sequences increase the likelihood of resistance emerging to the final drug when compared to giving that drug alone.
By checking all possible sequences of two, three or four antibiotics for which empirical landscapes are known, the research reveals that the majority, over 70%, of drug sequences increase the likelihood of resistance emerging to the final drug (when compared to giving that drug alone).
In particular, giving Piperacillin+Tazobactam, an antibiotic often used after others fail, as the final drug in a sequence of two or three antibiotics increases the likelihood of resistance arising in over 90% of cases. By giving drugs in arbitrary orders we may be inadvertently encouraging the emergence of antibiotic resistance just as giving drugs with incorrect doses can do so.
To move their theoretical findings towards the clinic, Daniel and Jacob have partnered with microbiologists at the Louis Stokes Department of Veterans Affairs Hospital in Cleveland to perform empirical tests of evolutionary steering. They are also working with Dr Alexander Anderson and Dr Robert Gatenby at the Moffitt Cancer Center, to adapt their model to predicting the effectiveness of cancer therapies.
A major impediment to designing adaptive therapies using their method is that measuring fitness landscapes is a complex problem where the number of strains that need to be synthesised grows exponentially with the number of mutations of interest. Despite this difficulty, empirical fitness landscape research, aided by machine learning techniques which help to reduce the number of strains that need to be synthesised, has grown rapidly in recent years. As this trend continues the potential for therapies exploiting evolutionary steering, and with it the role of computational models in designing treatment, will continue to grow - hopefully enabling better treatment for a variety of deadly diseases in the future.
The paper, Steering Evolution with Sequential Therapy to Prevent the Emergence of Bacterial Antibiotic Resistance, is published in PLOS Computational Biology (doi: 10.1371/journal.pcbi.1004493)
This blog originally appeared in Inspired Research (PDF), the magazine of Oxford University's Department of Computer Sciences