Helping the ‘Greeks’ to run faster
Mathematical techniques developed by Professor Mike Giles have led to substantial reductions in the complexity of the Monte Carlo computer simulations run by large banks, cutting both computing costs and energy consumption.
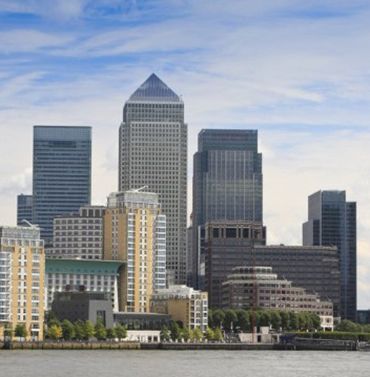
(Image credit: Shutterstock)
High performance computing is an integral part of modern investment banking. The largest investment banks in London each have tens of thousands of servers largely devoted to running simulations that compute what the industry calls ‘Greeks’: measures of risk that are used to estimate (and so minimise) possible future losses due to fluctuations in the market. The simulations use Monte Carlo methods, computational algorithms that rely on repeated random sampling to obtain information about the probability of certain events.
With such vast computer needs, the power consumption of financial data centres is a serious issue. In 2008, for example, the prioritising of the power infrastructure for the new Olympic Park in east London meant that the power companies were unable to provide cabling for any new financial data centres in the City. As a result, large banks were forced to build data centres beyond the M25 where power was available, or to buy in supercomputer services from IT providers at enormous cost.
Professor Mike Giles realised that a set of mathematical methods called adjoint techniques could be applied to the banks’ calculation of Greeks. Giles was a leading researcher in the use of adjoints in engineering design optimisation; the techniques make it possible to efficiently compute the sensitivity of a single output (such as the drag on a plane’s wing) to changes in the large number of design variables which determine its shape.
Giles saw that Greeks could be treated in a similar way, since financial markets also have a very large number of input variables that need to be taken into consideration when calculating risk. In 2006, together with Professor Paul Glasserman from Columbia University, he published the paper ‘Smoking adjoints: fast Monte Carlo Greeks’ in RISK, the leading publication for those working in quantitative finance.
The paper showed that it was possible to significantly improve the speed and accuracy of the banks’ computations, and was recognised immediately by the finance industry as a significant advance on the state-of-the-art. One bank in particular, Credit Suisse, was very quick to understand the potential of adjoint approaches and was able to develop them into a scalable risk management framework based on adjoint algorithmic differentiation, citing among its advantages:
- Considerable savings in computing costs
- Better risk management
- Enhanced profitability through improved hedging of risk
Around 20% of the Tier 1 banks are now thought to use adjoints on a daily basis as part of their routine option pricing processes, and most of the others are believed to be developing the technology. In practical terms, it means that banks can either reduce their computing costs and energy consumption, or increase the number of simulations they can afford to perform, leading to improved modelling and mitigation of risk. In the words of a trader at a European bank, “it means you can do far more simulations, for far more risk parameters, with far less computational cost.” This equates to a major reduction in cost as well as energy consumption – an environmental as well as an economic benefit.
Funded by the Oxford-Man Institute